
28 Apr An Introduction to Artificial Intelligence in Marketing via @jes_scholz
Marketing with Artificial Intelligence. Sounds fancy.
And that’s the problem.
The term is thrown around in marketing tool ad copy, by marketing gurus and hyped by the media.
Yet, a concrete definition is elusive.
For many, AI is an enigma surrounded by buzzwords.
But the irony is, as much as the hype has overstated what AI might do in the next years, the reality of how AI is already used today in marketing is often under-recognized.
- Facebook uses facial recognition to recommend who to tag in photos.
- Google uses deep learning to rank search results.
- Netflix uses machine learning to personalize recommendations.
- Amazon uses natural language processing for Alexa.
- The Washington Post uses natural language generation to write data-driven articles.
Your life is already machine-assisted, and your marketing can be, too.
What Is Artificial Intelligence?
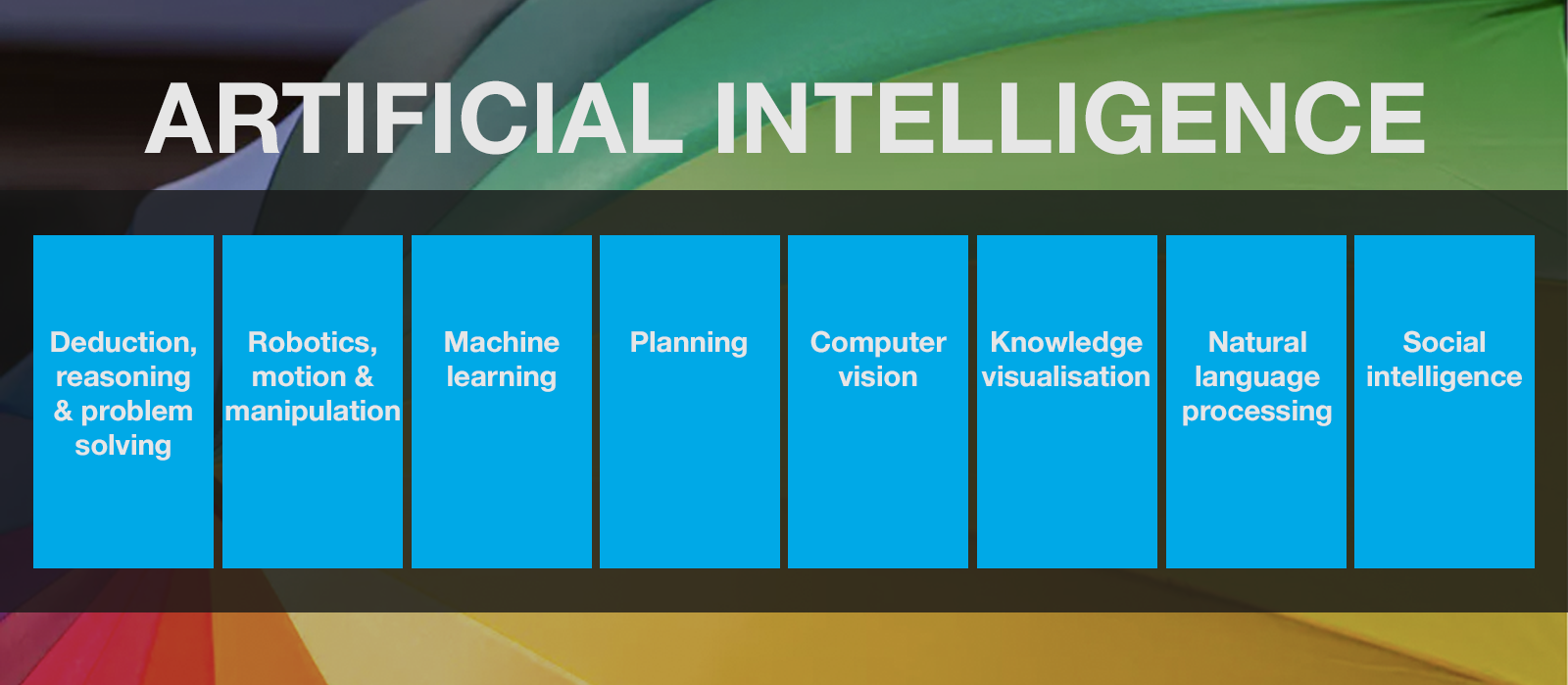
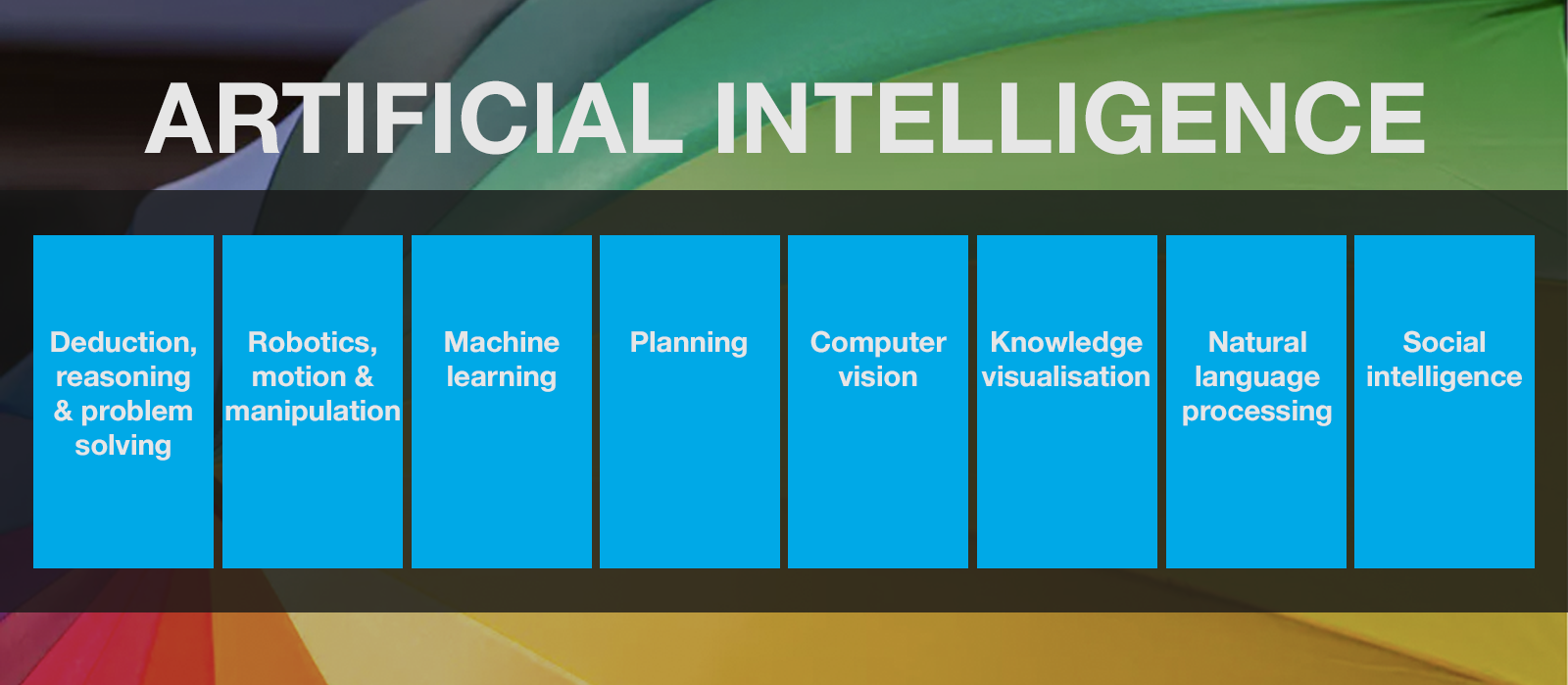
The best way to understand artificial intelligence is as an umbrella term.
It’s used to describe a suite of unique, but related, technologies that can simulate human capabilities.
It’s not some singular magic technology that can do everything.
But a set of individual tools with real capabilities, but which are at different stages of development.
Out of these different subsets of AI, there are a few that are particularly applicable to marketing right now.
- Computer vision which allows AI to see. This leads to object detection, facial recognition, and visual listening on social media.
- Natural language processing (NLP) which allows AI to hear and speak – giving us chatbots, semantic analysis, content generation, and voice search capabilities.
- Machine learning allows AI to learn with data on how to progressively improve performance on a specific task over time, without explicitly being programmed what to do. This gives us content recommendations, lookalike audiences, programmatic advertising, and lead scoring.
The ability of self-improvement provided by machine learning is the most critical subset of AI for marketers.
Automation Is Not Equal to Machine Learning
Maybe you’re thinking you’ve got this whole AI marketing thing covered because you have an expensive marketing automation tool.
Let’s get one thing crystal clear:
Automation is not machine learning.
Automation is a set of instructions that tells a machine what to do in order to produce a specified outcome.
You still have to design and input marketing logic.
Machine learning enables it to improve from experience, so the machine learns what to do in order to produce the desired outcome.
The machine is not restricted to pure execution, it also takes care of optimization.
At the core, automation replicates what you do now. It saves time but has little direct impact on KPIs.
On the other hand, machine learning will not only save you time but also improve on current tactics to continually drive up KPIs.
So why aren’t we leveraging this technology?
Let’s take a look at the top four reasons marketers are hesitant to adopt applications of AI and how to overcome them.
Reason 1: Lack of Technical Skills
Although many marketers feel they lack the technical skills to adopt AI.
This need not be the case.
The reality is, you already know everything you need to get started.
There is a difference between machine learning research, which is all about building better algorithms and is the prerogative of data scientists, and applied machine learning, which is using algorithms to solve business issues, which is what marketers need to do.
Think about it this way:
You may not fully understand the science behind how a microwave works. But that doesn’t stop you from using it to cook.


- Companies without data scientists can still choose the best data (best ingredients).
- Put this information into open-sourced algorithms (the appliance).
- To create models (the recipes).
- That produces predictions – a.k.a., enact marketing tactics (the dish).
- The quality of which we can assess by testing (tasting).
And if the dish isn’t tasty, you can modify the recipe, or buy a stove, or get better quality ingredients.
You won’t become a better chef by learning more about the science behind how a microwave works.
You won’t become a better marketer, by researching the intricacies of data science.
The best way to learn to cook is to just get started.
The best way for marketers to overcome our problem of scale is to roll out any use case of AI.
Reason 2: Fear of our Jobs
Some teams don’t want to begin AI initiatives as they fear it will cause the next industrial revolution and they will be out of a job – this naturally causes a lot of resistance.
Unless you plan to retire in the next 5 years, artificial intelligence will significantly impact your career in marketing. But this doesn’t mean you will be replaced by a marketing robot.
Your job will change from executing repetitive tasks to teaching AI to do those tasks for you.
Allowing you to reinvest your time into creativity and strategy.
But what does teaching an AI actually involve?
Because it sounds very technical.
The most common training method for marketing use cases is supervised learning.
This involves two phases.
The first is the initial teaching process.


Let’s say you have 1 million customer reviews.
No human could possibly read them all, so you want to use machine learning to understand the sentiment, classifying the review as positive, neutral, or negative.
To achieve this, take a sample of those reviews and label each of them with one of your three classifications.
Then feed this training data into your machine learning algorithm.
The more data it has, the better it will be at recognizing patterns and over time the more reliably it will be able to classify the sentiment of reviews on its own.
To test its abilities, rather than feeding it labeled data, input the raw data, and assess the quality of the results.
Often, if you have done the initial teaching process well, it will already be able to correctly classify a large portion of the data.
And you can move onto the second phase; the ongoing teaching process.


Where on a regular basis you would re-labeling any errors to teach the algorithm what it did wrong, allowing it to continually improve.
You may have already been teaching algorithms without knowing it.
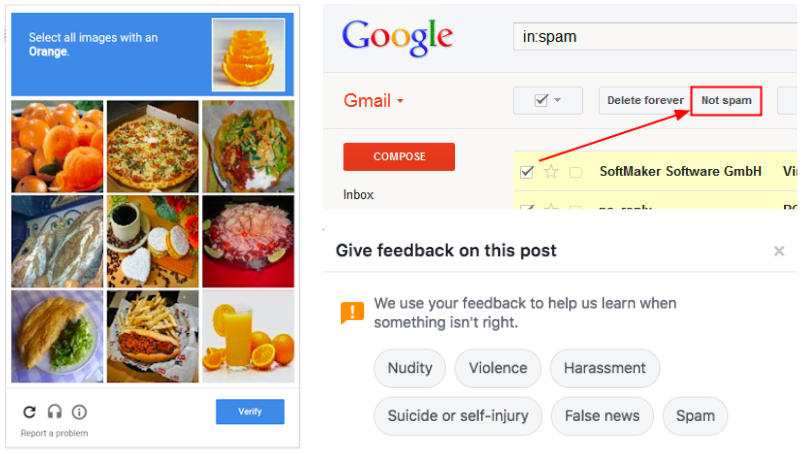
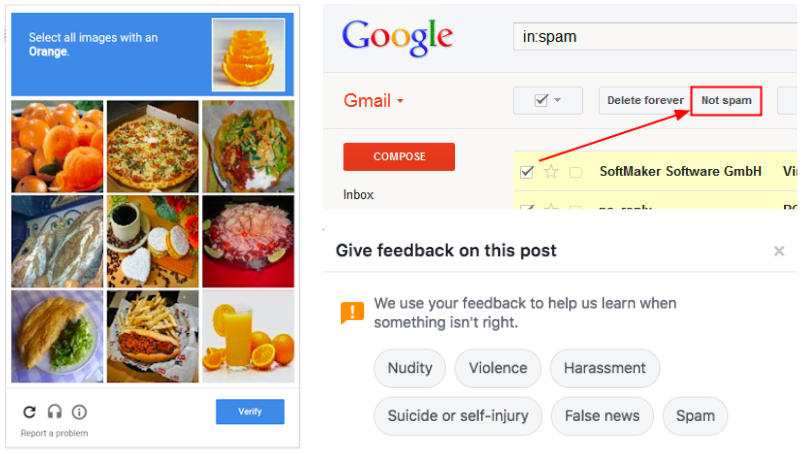
Who has filled in an image-based Google captcha, marked an email as not spam or marked fake news on a Facebook post.
By each of these actions, you were proving manual verification, adding labels, and teaching the algorithms.
Think of launching a machine learning algorithm like hiring a new junior marketer.
The day you onboard it, is the worst day it’s ever going to perform.
It will do the work, but it will make mistakes, so you need to supervise the results, correcting as needed.
The longer it works, the better it becomes and the more time you have to reinvest into scaling other marketing channels.
But unlike a human, machines are happy to do the same, very narrow job forever – whether that’s classifying reviews, adjusting ad bids, posting on social media, or forecasting growth.
You are not handing over control of marketing to a machine.
You are teaching them how to collect the information you need or how to execute a specific element of your marketing strategy.
And AI offers a whole new level of scale.
Classifying 1 million reviews is not a problem for a machine learning algorithm.
What’s more, because of this scale, it can produce insights that would otherwise not be available.
What you choose to do with those insights is what becomes critical to success.
Those strategic questions are where marketers should be spending time.
Reason 3: Investment of Resources & Budget
Executives are often concerned about the implementation efforts and costs for AI applications.
So the best place to start is not by asking for more budget and resources, but by asking yourself are you fully leveraging what you are already paying for?
Consider the AI capabilities of your current marketing tool set.
Marketing automation platforms like HubSpot, CRMs like SalesForce, and Advertising tools like Google Ads and Facebook Ads have all incorporated AI into their systems.
If you’re a customer of one of these solutions, their support teams can be a valuable resource to begin your organization’s AI implementation as you can learn from their insights and experience.
It’s a great way to start building up your team competency in AI applications for little to no additional cost.
And what about the AI capabilities of your current tech stack?
AI technologies are not channel-based, they are use case-based.
So if you have a recommendation engine running on your website, why not use this machine learning algorithm to improve the personalization for your email newsletter, push notifications, or chatbot content.
You can use these existing technologies as low investment proof of concept.
So by the time you are asking for additional resources and budgets, your executives are already fully onboard.
When you are looking for a new tool, beware of buzzwords.
Many AI solutions aren’t actually that intelligent. Even when there are the words “AI” or “machine learning” right there in the product description.
Some tools shamelessly use these terms to describe commonplace automation or targeting capabilities.
Now that you have a deeper understanding of what AI really is, put those sales guys through their paces.
If the vendor can’t explain how the AI works in detail, don’t buy it. If it seems too good to be true, don’t buy it.
Because, I’m sorry to say, there is no marketing AI platform that neatly bundles everything up into a single monthly subscription.
That’s because AI is narrow in its capabilities and must be purpose-built to solve one, well-defined problem.
This is why you need to choose the right use case first – as each use case will likely need its own tool.
But what you can do is begin to build your own artificial intelligence.
AI technology is becoming more affordable and accessible because companies like Google, Amazon, IBM, and SalesForce are offering their algorithms to the world.
Some third-party services are open-source, others are pay to play – but they all give a springboard from where you can customize your own solution.
Especially if they offer access to additional data sets to layer onto your own 1st party data, making your AI application more powerful.
Reason 4: Quality of Data Sources
The very best AI tools and talent in the world will not deliver results if you are missing the most critical component for machine learning – high-quality data to inform the learning algorithm.
Data quality is probably the single biggest challenge you will face when implementing AI.


As eMarketer notes, data is often old, or in silos or we just don’t have enough in the first place.
And we’re not dedicating resources to fix this.
The problem is, feeding bad data into a good machine learning algorithm won’t give the right answers.
Without this understanding that data is of critical importance, you are likely to blame the poor outcomes on the AI.
There are things we marketers should be doing to drive for actionable data.
- When was the last time you did a Google Analytics audit?
- Have you implemented structured markup and content tagging?
- Are you using remarketing scripts to collect more user data?
- Are you supporting the collection of data that can be used to identify users across devices and channels, like email addresses?
- Have you got your marketing tools integrated with your Data Management Platform (DMP)?
You need to focus on these areas now, because good AI marketing depends upon having actionable data that is structured, integrated through a common identifier, plentiful, and (most importantly) accurate.
Looking to the Future
Artificial intelligence is changing consumer behavior.
Consumers are hit with too much information every day.
They don’t want to spend time evaluating all the options. So they delegate.
Think about what is already controlled by AI.
- Social algorithms influence what brands we engage with.
- Digital maps determine what routes we take or suggest places nearby to visit.
- Recommendation engines shape awareness and influence purchases.
- An AI may even determine who you marry. Based on your swiping left and right, the algorithm in your dating app chooses your match.
Algorithms will become the new gatekeepers, through devices like digital personal assistants.
And the future of your business depends on your ability to influence the AI’s that make the recommendations to the humans, if not the whole decision.
Think about it. When a customer asks their Google Home or Alexa to “order me cookies” it’s the AI who decides on the brand.
You must understand how that decision is made. Treat algorithms like a new audience. Understand their needs.
Start marketing to machines with machines.
More Resources:
- Marketing & AI: Key Takeaways You Need to Know
- I, Search: How AI Will Transform the Landscape of SEO
- What Is Artificial Emotional Intelligence & How Does Emotion AI Work?
Image Credits
Featured & In-Post Images: Created by author, April 2020
All screenshots taken by author, April 2020
Sorry, the comment form is closed at this time.